How to Build a Winning Data Portfolio
Building a strong data portfolio is essential for showcasing your skills and differentiating yourself from others in the field. A well-curated portfolio demonstrates not only your technical abilities but also your capacity to think critically and solve real-world problems. Whether you’re just starting out in data science or looking to level up your career, creating a portfolio that stands out is key. Here’s a guide on how to build a compelling data portfolio from coursework to real-world projects.
A Foundation for Your Data Portfolio
When building a data portfolio, academic projects often serve as the starting point. These projects provide a solid foundation, as they typically focus on the application of core data concepts and problem-solving methodologies. Academic assignments, such as class projects, homework, or research papers, showcase your understanding of fundamental data science concepts, including statistical analysis, machine learning algorithms, data cleaning, and data visualisation.
Make sure to include projects that reflect a variety of techniques and skills. For example, you could showcase a project where you applied linear regression to predict outcomes, another one where you used clustering to find patterns, and a visualisation project to present insights clearly. It’s important to present these projects in a way that highlights the practical skills you’ve gained, especially if you can draw from your coursework to address real-world problems.
Showcasing Initiative with a Passion Project
While academic projects are important, personal projects demonstrate a deeper level of initiative and passion for data work. Personal projects give you the freedom to choose problems that excite you, whether they relate to your hobbies, social issues, or areas of professional interest. These projects showcase your ability to apply data skills in unstructured settings, reflecting creativity and the ability to think independently.
For instance, you could work on a data analysis project related to sports statistics, social media sentiment analysis, or even financial forecasting using open datasets. When choosing personal projects, try to focus on projects that align with your career goals. If you want to work in healthcare data, for example, work on a project analysing public health data or disease outbreak trends. The more your personal projects align with the industry or role you’re targeting, the more compelling they will be to potential employers.
Highlight Real-World Application of Skills
Real-world projects are the final piece of the puzzle for a strong data portfolio. Internships, freelance work, or any work experience where you’ve applied data analysis to solve real problems should be prominently displayed. These projects show data leaders that you can take theory into practice and deliver results in a professional setting. G one better and add the experience to your CV and professional profiles, such as LinkedIn.
If you’re early in your career and don’t have formal work experience, try to include any freelance or volunteer data work you’ve done for small businesses, non-profits, or local projects. The key here is demonstrating the impact of your work. Showcase how you helped an organisation save costs, increase efficiency, or improve decision-making through data-driven insights. Including measurable results in your portfolio will make a strong case for your ability to add value.
What Data Leaders Look for in a Portfolio
When data leaders review portfolios, they look for a few key elements: technical proficiency, problem-solving ability, communication skills, and a results-oriented approach. They want to see that you not only understand the technical tools and methodologies but also can apply them to real business challenges.
A great portfolio should:
- Demonstrate Technical Skills: Show proficiency with programming languages (like Python or R), data wrangling, machine learning, and data visualisation tools.
- Solve Real Problems: Your portfolio should highlight how you’ve used data to solve real-world issues. Include projects that reflect how your work helped drive insights, optimise processes, or improve decision-making.
- Be Well-Presented: Data leaders also appreciate clarity and well-organised portfolios. They want to easily understand the problem, your approach, and the results.
- Show Communication Skills: Clear communication is key. Showcase your ability to explain complex data insights in a digestible and actionable way.
Visualisation and Storytelling
Once you’ve gathered the projects for your portfolio, it’s essential to present them clearly and engagingly. This is where storytelling and data visualisation come into play. A good data portfolio doesn’t just present data, it tells a story.
Make sure your portfolio is structured to guide the viewer through your thought process, methodologies, and conclusions. Here are some tips to ensure your projects stand out:
- Create a Clear Narrative: Present each project as a story. Start by defining the problem, explaining how you approached it, and then showcasing the insights you gained. Conclude with the impact of your findings. This will help data leaders see the value you can bring to an organisation.
- Use Visualisations to Clarify Insights: Visualisations are key to making your findings accessible and engaging. Use charts, graphs, and other visuals to clearly communicate your results. Make sure your visuals are easy to read and effectively highlight the insights you want to convey.
- Keep it Simple: Avoid overcomplicating your presentations. While it's tempting to show off every analysis you did, choose the most meaningful insights and present them in a way that anyone can understand. Remember, data leaders are looking for clarity and insight, not complexity for complexity's sake.
Tailoring Your Portfolio to Your Audience
Lastly, tailoring your portfolio to the specific industry or role you’re targeting is crucial. If you’re applying for a data analyst position, highlight projects where you’ve used data manipulation and statistical analysis to drive insights. If you’re pursuing a data science role, include machine learning projects where you’ve built models and validated results. By focusing on projects that align with the job description, you’ll make your portfolio more relevant and compelling.
Building a Strong Data Portfolio
Building a winning data portfolio is an ongoing process that evolves with your skills and experience. By including academic, personal, and real-world projects (like those undertaken in the Nurture Programme), you can demonstrate a well-rounded skill set to data leaders. Make sure to focus on the impact of your work, communicate clearly, and present your projects in an engaging and digestible way. A great data portfolio is not just a collection of work, it’s a powerful tool that showcases your ability to think critically, solve problems, and drive results.
Don’t be scared of show casing old projects, it shows your progress and you can also return to them at a future date. This way they can grow and evolve with you and your skills!
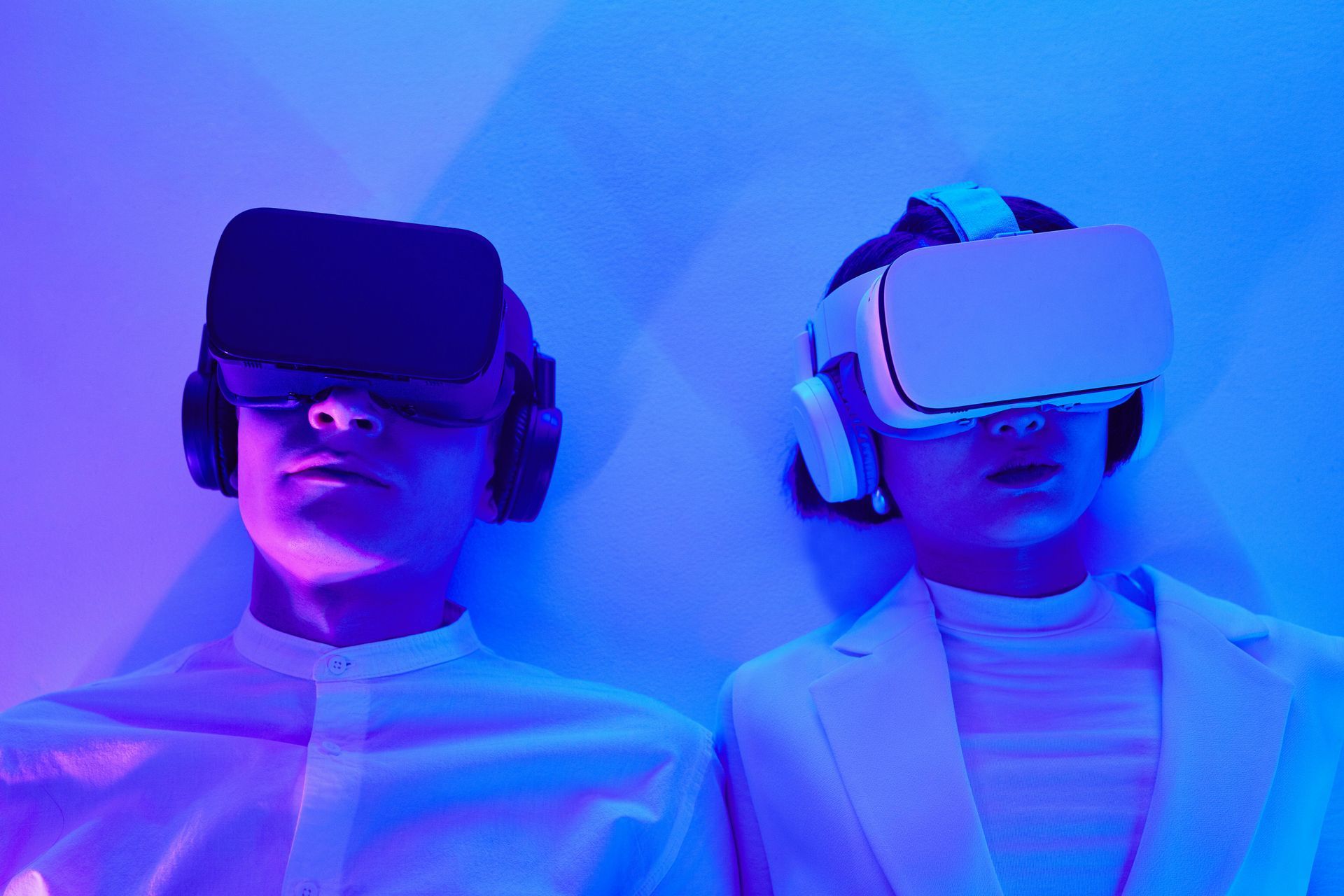
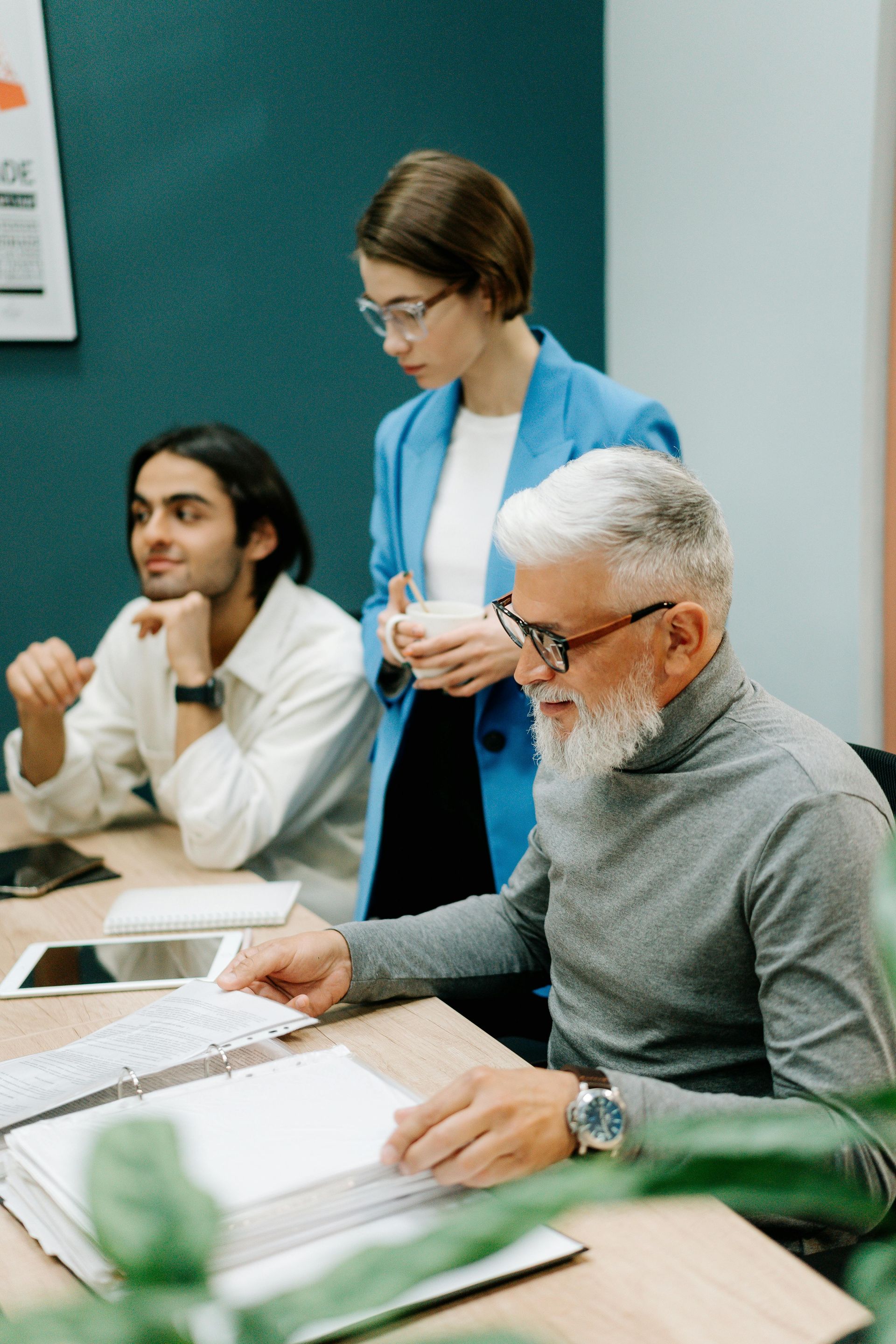
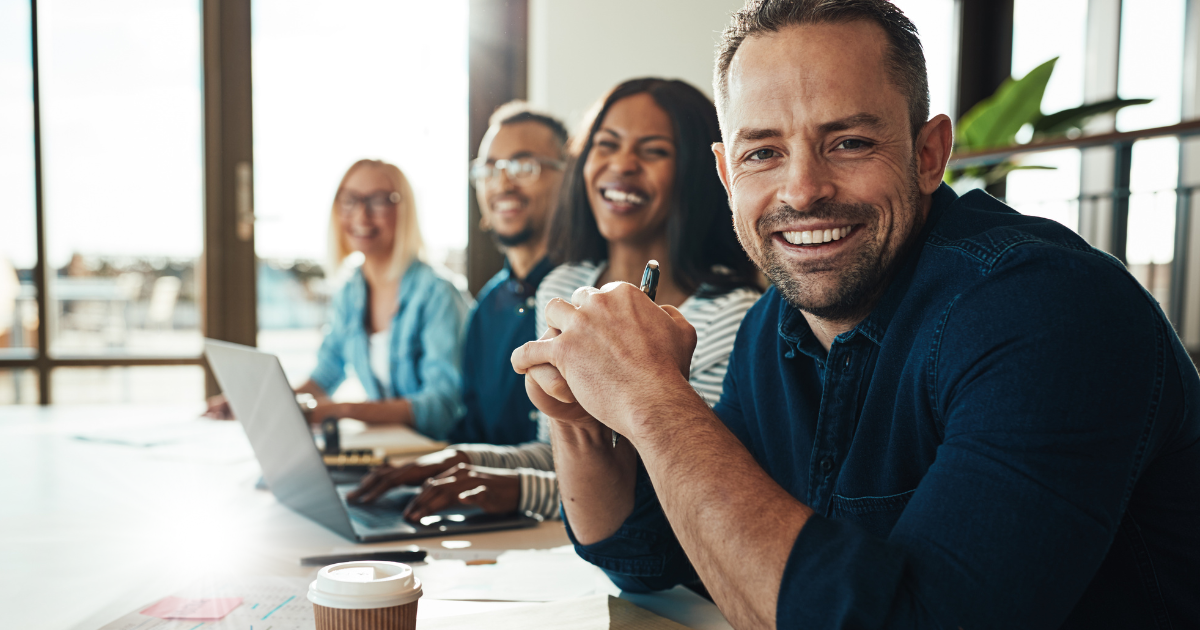
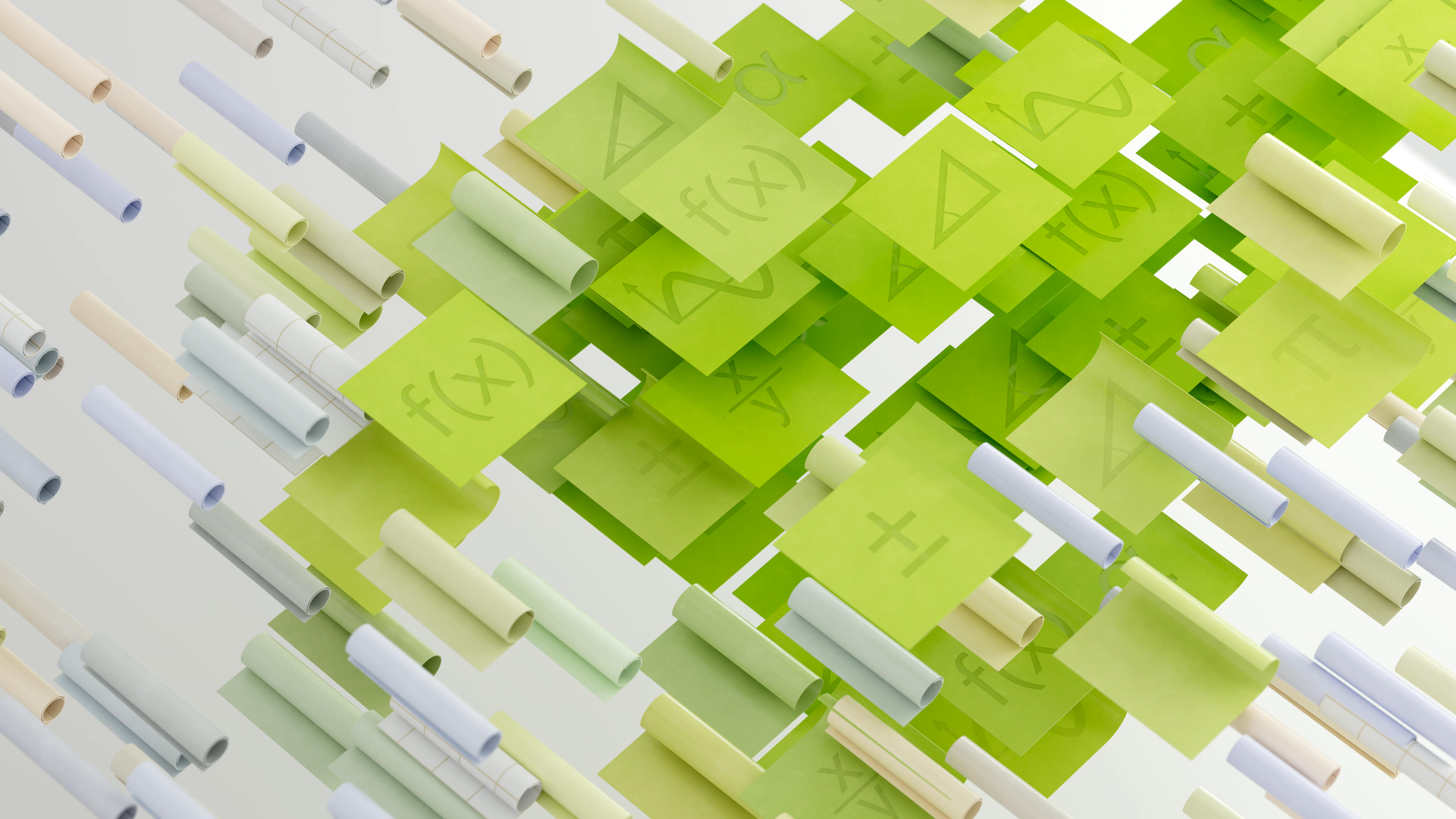
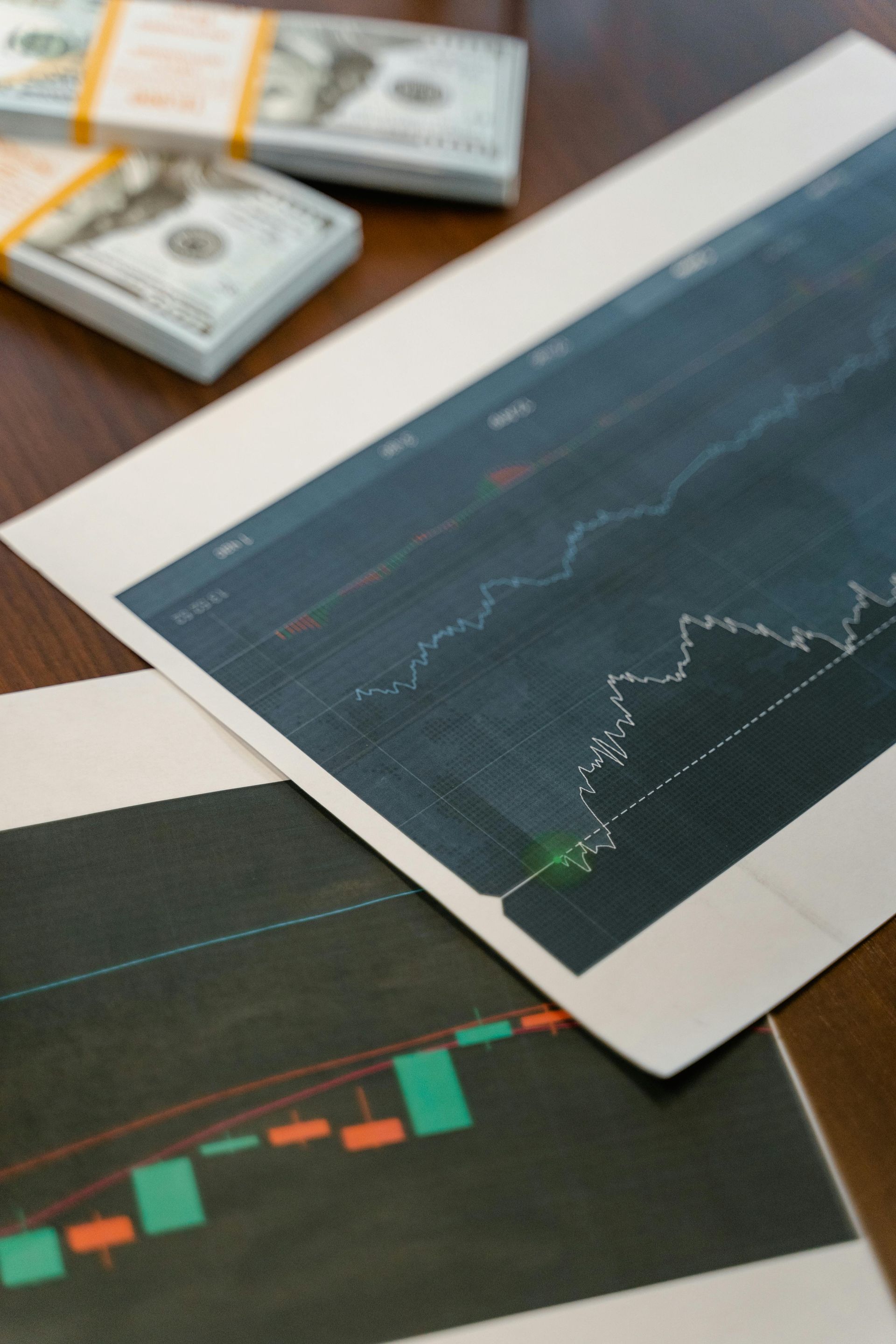